Deep Set Auto Encoders for Anomaly Detection in Particle Physics
Bryan Ostdiek
SciPost Phys. 12, 045 (2022) · published 31 January 2022
- doi: 10.21468/SciPostPhys.12.1.045
- Submissions/Reports
-
Abstract
There is an increased interest in model agnostic search strategies for physics beyond the standard model at the Large Hadron Collider. We introduce a Deep Set Variational Autoencoder and present results on the Dark Machines Anomaly Score Challenge. We find that the method attains the best anomaly detection ability when there is no decoding step for the network, and the anomaly score is based solely on the representation within the encoded latent space. This method was one of the top-performing models in the Dark Machines Challenge, both for the open data sets as well as the blinded data sets.
Cited by 21
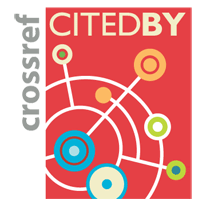
Author / Affiliations: mappings to Contributors and Organizations
See all Organizations.- 1 2 Bryan Ostdiek
- 1 Harvard University
- 2 The NSF AI Institute for Artificial Intelligence and Fundamental Interactions [IAIFI]
Funders for the research work leading to this publication