Symmetries, safety, and self-supervision
Barry M. Dillon, Gregor Kasieczka, Hans Olischlager, Tilman Plehn, Peter Sorrenson, Lorenz Vogel
SciPost Phys. 12, 188 (2022) · published 9 June 2022
- doi: 10.21468/SciPostPhys.12.6.188
- Submissions/Reports
-
Abstract
Collider searches face the challenge of defining a representation of high-dimensional data such that physical symmetries are manifest, the discriminating features are retained, and the choice of representation is new-physics agnostic. We introduce JetCLR to solve the mapping from low-level data to optimized observables though self-supervised contrastive learning. As an example, we construct a data representation for top and QCD jets using a permutation-invariant transformer-encoder network and visualize its symmetry properties. We compare the JetCLR representation with alternative representations using linear classifier tests and find it to work quite well.
Cited by 25
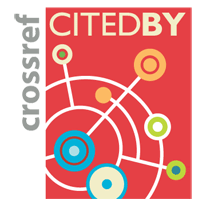
Authors / Affiliations: mappings to Contributors and Organizations
See all Organizations.- 1 Barry M. Dillon,
- 2 Gregor Kasieczka,
- 1 Hans Olischlager,
- 1 Tilman Plehn,
- 1 Peter Sorrenson,
- 1 Lorenz Vogel
- 1 Ruprecht-Karls-Universität Heidelberg / Heidelberg University
- 2 Universität Hamburg / University of Hamburg [UH]