Ephemeral Learning - Augmenting Triggers with Online-Trained Normalizing Flows
Anja Butter, Sascha Diefenbacher, Gregor Kasieczka, Benjamin Nachman, Tilman Plehn, David Shih, Ramon Winterhalder
SciPost Phys. 13, 087 (2022) · published 7 October 2022
- doi: 10.21468/SciPostPhys.13.4.087
- Submissions/Reports
-
Abstract
The large data rates at the LHC require an online trigger system to select relevant collisions. Rather than compressing individual events, we propose to compress an entire data set at once. We use a normalizing flow as a deep generative model to learn the probability density of the data online. The events are then represented by the generative neural network and can be inspected offline for anomalies or used for other analysis purposes. We demonstrate our new approach for a toy model and a correlation-enhanced bump hunt.
Cited by 9
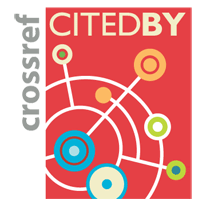
Authors / Affiliations: mappings to Contributors and Organizations
See all Organizations.- 1 2 Anja Butter,
- 3 Sascha Diefenbacher,
- 3 Gregor Kasieczka,
- 4 5 Benjamin Nachman,
- 1 Tilman Plehn,
- 6 David Shih,
- 7 Ramon Winterhalder
- 1 Ruprecht-Karls-Universität Heidelberg / Heidelberg University
- 2 Sorbonne Université / Sorbonne University
- 3 Universität Hamburg / University of Hamburg [UH]
- 4 University of California, Berkeley [UCBL]
- 5 Lawrence Berkeley National Laboratory [LBNL]
- 6 Rutgers University [RU]
- 7 Université catholique de Louvain [UCL]
Funders for the research work leading to this publication
- Australian Research Council [ARC]
- Deutsche Forschungsgemeinschaft / German Research FoundationDeutsche Forschungsgemeinschaft [DFG]
- Fonds De La Recherche Scientifique - FNRS (FNRS) (through Organization: Fonds National de la Recherche Scientifique [FNRS])
- United States Department of Energy [DOE]