How to understand limitations of generative networks
Ranit Das, Luigi Favaro, Theo Heimel, Claudius Krause, Tilman Plehn, David Shih
SciPost Phys. 16, 031 (2024) · published 25 January 2024
- doi: 10.21468/SciPostPhys.16.1.031
- Submissions/Reports
-
Abstract
Well-trained classifiers and their complete weight distributions provide us with a well-motivated and practicable method to test generative networks in particle physics. We illustrate their benefits for distribution-shifted jets, calorimeter showers, and reconstruction-level events. In all cases, the classifier weights make for a powerful test of the generative network, identify potential problems in the density estimation, relate them to the underlying physics, and tie in with a comprehensive precision and uncertainty treatment for generative networks.
Cited by 12
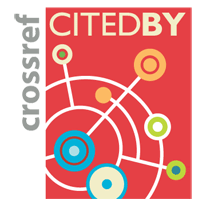
Authors / Affiliations: mappings to Contributors and Organizations
See all Organizations.- 1 Ranit Das,
- 2 Luigi Favaro,
- 2 Theo Heimel,
- 2 Claudius Krause,
- 2 Tilman Plehn,
- 1 David Shih
Funders for the research work leading to this publication