GANplifying event samples
Anja Butter, Sascha Diefenbacher, Gregor Kasieczka, Benjamin Nachman, Tilman Plehn
SciPost Phys. 10, 139 (2021) · published 10 June 2021
- doi: 10.21468/SciPostPhys.10.6.139
- Submissions/Reports
-
Abstract
A critical question concerning generative networks applied to event generation in particle physics is if the generated events add statistical precision beyond the training sample. We show for a simple example with increasing dimensionality how generative networks indeed amplify the training statistics. We quantify their impact through an amplification factor or equivalent numbers of sampled events.
Cited by 43
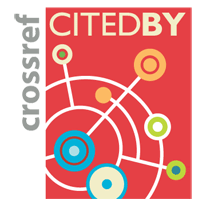
Authors / Affiliations: mappings to Contributors and Organizations
See all Organizations.- 1 Ruprecht-Karls-Universität Heidelberg / Heidelberg University
- 2 Universität Hamburg / University of Hamburg [UH]
- 3 Lawrence Berkeley National Laboratory [LBNL]
Funders for the research work leading to this publication