Learning crystal field parameters using convolutional neural networks
Noah F. Berthusen, Yuriy Sizyuk, Mathias S. Scheurer, Peter P. Orth
SciPost Phys. 11, 011 (2021) · published 14 July 2021
- doi: 10.21468/SciPostPhys.11.1.011
- Submissions/Reports
-
Abstract
We present a deep machine learning algorithm to extract crystal field (CF) Stevens parameters from thermodynamic data of rare-earth magnetic materials. The algorithm employs a two-dimensional convolutional neural network (CNN) that is trained on magnetization, magnetic susceptibility and specific heat data that is calculated theoretically within the single-ion approximation and further processed using a standard wavelet transformation. We apply the method to crystal fields of cubic, hexagonal and tetragonal symmetry and for both integer and half-integer total angular momentum values $J$ of the ground state multiplet. We evaluate its performance on both theoretically generated synthetic and previously published experimental data on CeAgSb$_2$, PrAgSb$_2$ and PrMg$_2$Cu$_9$, and find that it can reliably and accurately extract the CF parameters for all site symmetries and values of $J$ considered. This demonstrates that CNNs provide an unbiased approach to extracting CF parameters that avoids tedious multi-parameter fitting procedures.
Cited by 2
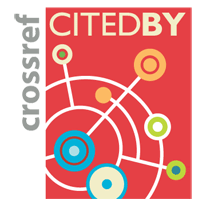
Authors / Affiliations: mappings to Contributors and Organizations
See all Organizations.- 1 2 Noah F. Berthusen,
- 1 2 Yuriy Sizyuk,
- 3 4 Mathias S. Scheurer,
- 1 2 Peter P. Orth
- 1 Iowa State University [ISU]
- 2 Ames Laboratory
- 3 Universität Innsbruck / University of Innsbruck [UBI]
- 4 Harvard University