Picking the low-hanging fruit: testing new physics at scale with active learning
Juan Rocamonde, Louie Corpe, Gustavs Zilgalvis, Maria Avramidou, Jon Butterworth
SciPost Phys. 13, 002 (2022) · published 15 July 2022
- doi: 10.21468/SciPostPhys.13.1.002
- Submissions/Reports
-
Abstract
Since the discovery of the Higgs boson, testing the many possible extensions to the Standard Model has become a key challenge in particle physics. This paper discusses a new method for predicting the compatibility of new physics theories with existing experimental data from particle colliders. By using machine learning, the technique obtained comparable results to previous methods (>90% precision and recall) with only a fraction of their computing resources (<10%). This makes it possible to test models that were impossible to probe before, and allows for large-scale testing of new physics theories.
Cited by 4
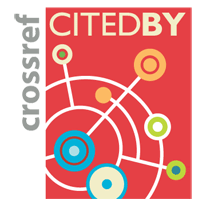
Authors / Affiliations: mappings to Contributors and Organizations
See all Organizations.- 1 Juan Rocamonde,
- 2 Louie Corpe,
- 1 G. Zilgalvis,
- 3 Maria Avramidou,
- 3 Jonathan Mark Butterworth
- 1 University of Cambridge
- 2 Organisation européenne pour la recherche nucléaire / European Organization for Nuclear Research [CERN]
- 3 University College London [UCL]
- Horizon 2020 (through Organization: European Commission [EC])