$\nu$-flows: Conditional neutrino regression
Matthew Leigh, John Andrew Raine, Knut Zoch, Tobias Golling
SciPost Phys. 14, 159 (2023) · published 16 June 2023
- doi: 10.21468/SciPostPhys.14.6.159
- Submissions/Reports
-
Abstract
We present $\nu$-Flows, a novel method for restricting the likelihood space of neutrino kinematics in high-energy collider experiments using conditional normalising flows and deep invertible neural networks. This method allows the recovery of the full neutrino momentum which is usually left as a free parameter and permits one to sample neutrino values under a learned conditional likelihood given event observations. We demonstrate the success of $\nu$-Flows in a case study by applying it to simulated semileptonic $t\bar{t}$ events and show that it can lead to more accurate momentum reconstruction, particularly of the longitudinal coordinate. We also show that this has direct benefits in a downstream task of jet association, leading to an improvement of up to a factor of 1.41 compared to conventional methods.
Cited by 3
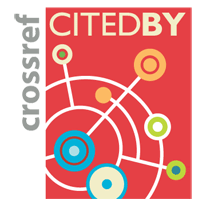
Authors / Affiliation: mappings to Contributors and Organizations
See all Organizations.- 1 Matthew Leigh,
- 1 John Andrew Raine,
- 1 Knut Zoch,
- 1 Tobias Golling