Accelerating equilibrium spin-glass simulations using quantum annealers via generative deep learning
Giuseppe Scriva, Emanuele Costa, Benjamin McNaughton, Sebastiano Pilati
SciPost Phys. 15, 018 (2023) · published 20 July 2023
- doi: 10.21468/SciPostPhys.15.1.018
- Submissions/Reports
-
Abstract
Adiabatic quantum computers, such as the quantum annealers commercialized by D-Wave Systems Inc., are routinely used to tackle combinatorial optimization problems. In this article, we show how to exploit them to accelerate equilibrium Markov chain Monte Carlo simulations of computationally challenging spin-glass models at low but finite temperatures. This is achieved by training generative neural networks on data produced by a D-Wave quantum annealer, and then using them to generate smart proposals for the Metropolis-Hastings algorithm. In particular, we explore hybrid schemes by combining single spin-flip and neural proposals, as well as D-Wave and classical Monte Carlo training data. The hybrid algorithm outperforms the single spin-flip Metropolis-Hastings algorithm. It is competitive with parallel tempering in terms of correlation times, with the significant benefit of a much shorter equilibration time.
Cited by 1
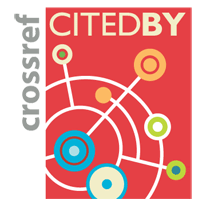
Authors / Affiliations: mappings to Contributors and Organizations
See all Organizations.- 1 2 Giuseppe Scriva,
- 1 2 Emanuele Costa,
- 2 3 Benjamin McNaughton,
- 1 2 Sebastiano Pilati
- 1 INFN Sezione di Perugia
- 2 Università degli Studi di Camerino / University of Camerino
- 3 Universiteit Antwerpen / University of Antwerp
- Horizon 2020 (through Organization: European Commission [EC])
- Ministero dell’Istruzione, dell’Università e della Ricerca (MIUR) (through Organization: Ministero dell'Istruzione, dell'Università e della Ricerca / Ministry of Education, Universities and Research [MIUR])
- Partnership for Advanced Computing in Europe AISBL (PRACE) (through Organization: Partnership for Advanced Computing in Europe [PRACE])