Generative invertible quantum neural networks
Armand Rousselot, Michael Spannowsky
SciPost Phys. 16, 146 (2024) · published 4 June 2024
- doi: 10.21468/SciPostPhys.16.6.146
- Submissions/Reports
-
Abstract
Invertible Neural Networks (INN) have become established tools for the simulation and generation of highly complex data. We propose a quantum-gate algorithm for a Quantum Invertible Neural Network (QINN) and apply it to the LHC data of jet-associated production of a Z-boson that decays into leptons, a standard candle process for particle collider precision measurements. We compare the QINN's performance for different loss functions and training scenarios. For this task, we find that a hybrid QINN matches the performance of a significantly larger purely classical INN in learning and generating complex data.
Cited by 2
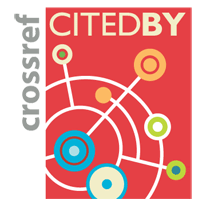