Deep-learning jets with uncertainties and more
Sven Bollweg, Manuel Haussmann, Gregor Kasieczka, Michel Luchmann, Tilman Plehn, Jennifer Thompson
SciPost Phys. 8, 006 (2020) · published 16 January 2020
- doi: 10.21468/SciPostPhys.8.1.006
- Submissions/Reports
-
Abstract
Bayesian neural networks allow us to keep track of uncertainties, for example in top tagging, by learning a tagger output together with an error band. We illustrate the main features of Bayesian versions of established deep-learning taggers. We show how they capture statistical uncertainties from finite training samples, systematics related to the jet energy scale, and stability issues through pile-up. Altogether, Bayesian networks offer many new handles to understand and control deep learning at the LHC without introducing a visible prior effect and without compromising the network performance.
Cited by 34
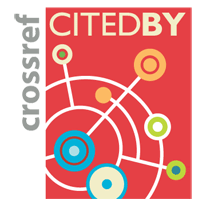
Ontology / Topics
See full Ontology or Topics database.Authors / Affiliations: mappings to Contributors and Organizations
See all Organizations.- 1 Sven Bollweg,
- 2 Manuel Haussmann,
- 1 Gregor Kasieczka,
- 2 Michel Luchmann,
- 2 Tilman Plehn,
- 2 Jennifer Thompson
- 1 Universität Hamburg / University of Hamburg [UH]
- 2 Ruprecht-Karls-Universität Heidelberg / Heidelberg University