On the descriptive power of Neural-Networks as constrained Tensor Networks with exponentially large bond dimension
Mario Collura, Luca Dell'Anna, Timo Felser, Simone Montangero
SciPost Phys. Core 4, 001 (2021) · published 2 February 2021
- doi: 10.21468/SciPostPhysCore.4.1.001
- Submissions/Reports
-
Abstract
In many cases, Neural networks can be mapped into tensor networks with an exponentially large bond dimension. Here, we compare different sub-classes of neural network states, with their mapped tensor network counterpart for studying the ground state of short-range Hamiltonians. We show that when mapping a neural network, the resulting tensor network is highly constrained and thus the neural network states do in general not deliver the naive expected drastic improvement against the state-of-the-art tensor network methods. We explicitly show this result in two paradigmatic examples, the 1D ferromagnetic Ising model and the 2D antiferromagnetic Heisenberg model, addressing the lack of a detailed comparison of the expressiveness of these increasingly popular, variational ans\"atze.
Cited by 6
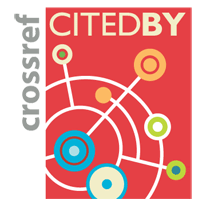
Authors / Affiliations: mappings to Contributors and Organizations
See all Organizations.- 1 2 3 Mario Collura,
- 2 Luca Dell'Anna,
- 2 3 4 Timo Felser,
- 2 4 Simone Montangero
- 1 Scuola Internazionale Superiore di Studi Avanzati / International School for Advanced Studies [SISSA]
- 2 Università degli Studi di Padova / University of Padua [UNIPD]
- 3 Universität des Saarlandes / Saarland University
- 4 Istituto Nazionale di Fisica Nucleare / National Institute for Nuclear Physics [INFN]
- Bundesministerium für Bildung und Forschung / Federal Ministry of Education and Research [BMBF]
- Deutsche Forschungsgemeinschaft / German Research FoundationDeutsche Forschungsgemeinschaft [DFG]
- European Commission [EC]
- Ministero dell’Istruzione, dell’Università e della Ricerca (MIUR) (through Organization: Ministero dell'Istruzione, dell'Università e della Ricerca / Ministry of Education, Universities and Research [MIUR])