Accelerating Monte Carlo event generation -- rejection sampling using neural network event-weight estimates
Katharina Danziger, Timo Janßen, Steffen Schumann, Frank Siegert
SciPost Phys. 12, 164 (2022) · published 18 May 2022
- doi: 10.21468/SciPostPhys.12.5.164
- Submissions/Reports
-
Abstract
The generation of unit-weight events for complex scattering processes presents a severe challenge to modern Monte Carlo event generators. Even when using sophisticated phase-space sampling techniques adapted to the underlying transition matrix elements, the efficiency for generating unit-weight events from weighted samples can become a limiting factor in practical applications. Here we present a novel two-staged unweighting procedure that makes use of a neural-network surrogate for the full event weight. The algorithm can significantly accelerate the unweighting process, while it still guarantees unbiased sampling from the correct target distribution. We apply, validate and benchmark the new approach in high-multiplicity LHC production processes, including $Z/W$+4 jets and $t\bar{t}$+3 jets, where we find speed-up factors up to ten.
Cited by 18
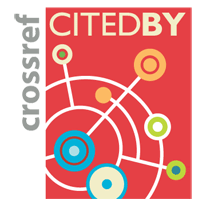
Authors / Affiliations: mappings to Contributors and Organizations
See all Organizations.- 1 Katharina Danziger,
- 2 Timo Janßen,
- 2 Steffen Schumann,
- 1 Frank Siegert
- 1 Technische Universität Dresden / Dresden University of Technology [TUD]
- 2 Georg-August-Universität Göttingen / University of Göttingen [GAU]