Modeling hadronization using machine learning
Phil Ilten, Tony Menzo, Ahmed Youssef, Jure Zupan
SciPost Phys. 14, 027 (2023) · published 2 March 2023
- doi: 10.21468/SciPostPhys.14.3.027
- Submissions/Reports
-
Abstract
We present the first steps in the development of a new class of hadronization models utilizing machine learning techniques. We successfully implement, validate, and train a conditional sliced-Wasserstein autoencoder to replicate the Pythia generated kinematic distributions of first-hadron emissions, when the Lund string model of hadronization implemented in Pythia is restricted to the emissions of pions only. The trained models are then used to generate the full hadronization chains, with an IR cutoff energy imposed externally. The hadron multiplicities and cumulative kinematic distributions are shown to match the Pythia generated ones. We also discuss possible future generalizations of our results.
Cited by 5
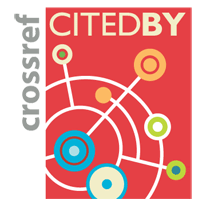
Authors / Affiliation: mappings to Contributors and Organizations
See all Organizations.- 1 Philip Ilten,
- 1 Tony Menzo,
- 1 Ahmed Youssef,
- 1 Jure Zupan