Generative networks for precision enthusiasts
Anja Butter, Theo Heimel, Sander Hummerich, Tobias Krebs, Tilman Plehn, Armand Rousselot, Sophia Vent
SciPost Phys. 14, 078 (2023) · published 20 April 2023
- doi: 10.21468/SciPostPhys.14.4.078
- Submissions/Reports
-
Abstract
Generative networks are opening new avenues in fast event generation for the LHC. We show how generative flow networks can reach percent-level precision for kinematic distributions, how they can be trained jointly with a discriminator, and how this discriminator improves the generation. Our joint training relies on a novel coupling of the two networks which does not require a Nash equilibrium. We then estimate the generation uncertainties through a Bayesian network setup and through conditional data augmentation, while the discriminator ensures that there are no systematic inconsistencies compared to the training data.
Cited by 22
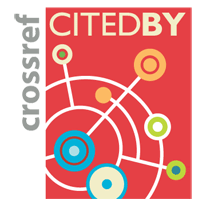
Authors / Affiliation: mappings to Contributors and Organizations
See all Organizations.- 1 Anja Butter,
- 1 Theo Heimel,
- 1 Sander Hummerich,
- 1 Tobias Krebs,
- 1 Tilman Plehn,
- 1 Armand Rousselot,
- 1 Sophia Vent