Autoregressive neural Slater-Jastrow ansatz for variational Monte Carlo simulation
Stephan Humeniuk, Yuan Wan, Lei Wang
SciPost Phys. 14, 171 (2023) · published 27 June 2023
- doi: 10.21468/SciPostPhys.14.6.171
- Submissions/Reports
-
Abstract
Direct sampling from a Slater determinant is combined with an autoregressive deep neural network as a Jastrow factor into a fully autoregressive Slater-Jastrow ansatz for variational quantum Monte Carlo, which allows for uncorrelated sampling. The elimination of the autocorrelation time leads to a stochastic algorithm with provable cubic scaling (with a potentially large prefactor), i.e. the number of operations for producing an uncorrelated sample and for calculating the local energy scales like $\mathcal{O}(N_s^3)$ with the number of orbitals $N_s$. The implementation is benchmarked on the two-dimensional $t-V$ model of spinless fermions on the square lattice.
Cited by 3
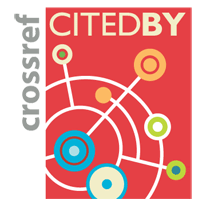
Authors / Affiliations: mappings to Contributors and Organizations
See all Organizations.- 1 2 Stephan Humeniuk,
- 1 3 Yuan Wan,
- 1 3 Lei Wang
- 1 中国科学院 / Chinese Academy of Sciences [CAS]
- 2 Rutgers University [RU]
- 3 松山湖材料实验室 / Songshan Lake Materials Laboratory [SLAB]