QCD or what?
Theo Heimel, Gregor Kasieczka, Tilman Plehn, Jennifer M Thompson
SciPost Phys. 6, 030 (2019) · published 8 March 2019
- doi: 10.21468/SciPostPhys.6.3.030
- Submissions/Reports
-
Abstract
Autoencoder networks, trained only on QCD jets, can be used to search for anomalies in jet-substructure. We show how, based either on images or on 4-vectors, they identify jets from decays of arbitrary heavy resonances. To control the backgrounds and the underlying systematics we can de-correlate the jet mass using an adversarial network. Such an adversarial autoencoder allows for a general and at the same time easily controllable search for new physics. Ideally, it can be trained and applied to data in the same phase space region, allowing us to efficiently search for new physics using un-supervised learning.
TY - JOUR
PB - SciPost Foundation
DO - 10.21468/SciPostPhys.6.3.030
TI - QCD or what?
PY - 2019/03/08
UR - https://scipost.org/SciPostPhys.6.3.030
JF - SciPost Physics
JA - SciPost Phys.
VL - 6
IS - 3
SP - 030
A1 - Heimel, Theo
AU - Kasieczka, Gregor
AU - Plehn, Tilman
AU - Thompson, Jennifer
AB - Autoencoder networks, trained only on QCD jets, can be used to search for anomalies in jet-substructure. We show how, based either on images or on 4-vectors, they identify jets from decays of arbitrary heavy resonances. To control the backgrounds and the underlying systematics we can de-correlate the jet mass using an adversarial network. Such an adversarial autoencoder allows for a general and at the same time easily controllable search for new physics. Ideally, it can be trained and applied to data in the same phase space region, allowing us to efficiently search for new physics using un-supervised learning.
ER -
@Article{10.21468/SciPostPhys.6.3.030,
title={{QCD or what?}},
author={Theo Heimel and Gregor Kasieczka and Tilman Plehn and Jennifer M Thompson},
journal={SciPost Phys.},
volume={6},
pages={030},
year={2019},
publisher={SciPost},
doi={10.21468/SciPostPhys.6.3.030},
url={https://scipost.org/10.21468/SciPostPhys.6.3.030},
}
Cited by 141
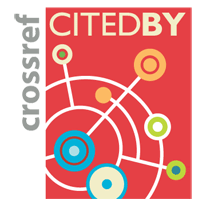
-
Komiske et al., Exploring the space of jets with CMS open data
Phys. Rev. D 101, 034009 (2020) [Crossref] -
Komiske et al., The hidden geometry of particle collisions
J. High Energ. Phys. 2020, 6 (2020) [Crossref] -
Andreassen et al., Simulation assisted likelihood-free anomaly detection
Phys. Rev. D 101, 095004 (2020) [Crossref] -
Ngairangbam et al., Anomaly detection in high-energy physics using a quantum autoencoder
Phys. Rev. D 105, 095004 (2022) [Crossref] -
Bhardwaj et al., Equivariant, safe and sensitive — graph networks for new physics
J. High Energ. Phys. 2024, 245 (2024) [Crossref] -
Kasieczka et al., The LHC Olympics 2020 a community challenge for anomaly detection in high energy physics
Rep. Prog. Phys. 84, 124201 (2021) [Crossref] -
Moreno et al., Interaction networks for the identification of boosted
H→bb¯
decays
Phys. Rev. D 102, 012010 (2020) [Crossref] -
Dolan et al., Metalearning and data augmentation for mass-generalized jet taggers
Phys. Rev. D 105, 094030 (2022) [Crossref] -
Dillon et al., Symmetries, safety, and self-supervision
SciPost Phys. 12, 188 (2022) [Crossref] -
Komiske et al., Metric Space of Collider Events
Phys. Rev. Lett. 123, 041801 (2019) [Crossref] -
Buhmann et al., Getting High: High Fidelity Simulation of High Granularity Calorimeters with High Speed
Comput Softw Big Sci 5, 13 (2021) [Crossref] -
Kuzu et al., Enhancing precision in J/ψ mass estimation: A study of ensemble and deep learning methods
Computer Physics Communications 310, 109534 109534 (2025) [Crossref] -
Bhardwaj et al., Foundations of automatic feature extraction at LHC–point clouds and graphs
Eur. Phys. J. Spec. Top. 233, 2619 (2024) [Crossref] -
Belis et al., Quantum anomaly detection in the latent space of proton collision events at the LHC
Commun Phys 7, 334 (2024) [Crossref] -
Bardhan et al., Searching for dark jets with displaced vertices using weakly supervised machine learning
Phys. Rev. D 108, 035036 (2023) [Crossref] -
Cerri et al., Variational autoencoders for new physics mining at the Large Hadron Collider
J. High Energ. Phys. 2019, 36 (2019) [Crossref] -
Gonski et al., High-dimensional anomaly detection with radiative return in e+e− collisions
J. High Energ. Phys. 2022, 156 (2022) [Crossref] -
Yalcin Kuzu, Evaluation of gradient boosting and deep learning algorithms in dimuon production
Journal of Molecular Structure 1277, 134834 134834 (2023) [Crossref] -
Ahmed et al., Application of deep learning in top pair and single top quark production at the LHC
Eur. Phys. J. Plus 138, 795 (2023) [Crossref] -
Batson et al., Topological obstructions to autoencoding
J. High Energ. Phys. 2021, 280 (2021) [Crossref] -
Bai et al., Non-resonant anomaly detection with background extrapolation
J. High Energ. Phys. 2024, 59 (2024) [Crossref] -
Aad et al., Dijet Resonance Search with Weak Supervision Using
s=13 TeV
pp
Collisions in the ATLAS Detector
Phys. Rev. Lett. 125, 131801 (2020) [Crossref] -
Collins et al., Comparing weak- and unsupervised methods for resonant anomaly detection
Eur. Phys. J. C 81, 617 (2021) [Crossref] -
Karagiorgi et al., Machine learning in the search for new fundamental physics
Nat Rev Phys 4, 399 (2022) [Crossref] -
Canelli et al., Autoencoders for semivisible jet detection
J. High Energ. Phys. 2022, 74 (2022) [Crossref] -
Farina et al., Searching for new physics with deep autoencoders
Phys. Rev. D 101, 075021 (2020) [Crossref] -
Larkoski et al., A theory of quark vs. gluon discrimination
J. High Energ. Phys. 2019, 14 (2019) [Crossref] -
Bradshaw et al., Mass agnostic jet taggers
SciPost Phys. 8, 011 (2020) [Crossref] -
et al., Search for periodic signals in the dielectron and diphoton invariant mass spectra using 139 fb−1 of pp collisions at $$ \sqrt{s} $$ = 13 TeV with the ATLAS detector
J. High Energ. Phys. 2023, 79 (2023) [Crossref] -
Atkinson et al., Anomaly detection with convolutional Graph Neural Networks
J. High Energ. Phys. 2021, 80 (2021) [Crossref] -
Grönroos et al., Automated visual inspection of CMS HGCAL silicon sensor surface using an ensemble of a deep convolutional autoencoder and classifier
Mach. Learn.: Sci. Technol. 4, 035028 (2023) [Crossref] -
Krupa et al., GPU coprocessors as a service for deep learning inference in high energy physics
Mach. Learn.: Sci. Technol. 2, 035005 (2021) [Crossref] -
Favaro et al., Semi-visible jets, energy-based models, and self-supervision
SciPost Phys. 18, 042 (2025) [Crossref] -
Park et al., Quasi anomalous knowledge: searching for new physics with embedded knowledge
J. High Energ. Phys. 2021, 30 (2021) [Crossref] -
Aguilar-Saavedra et al., Jet tagging made easy
Eur. Phys. J. C 80, 530 (2020) [Crossref] -
Finke et al., Autoencoders for unsupervised anomaly detection in high energy physics
J. High Energ. Phys. 2021, 161 (2021) [Crossref] -
Sahu, Integrating physics inspired features with graph convolution
Phys. Rev. D 111, 036037 (2025) [Crossref] -
Murphy, Class imbalance techniques for high energy physics
SciPost Phys. 7, 076 (2019) [Crossref] -
van Beekveld et al., Combining outlier analysis algorithms to identify new physics at the LHC
J. High Energ. Phys. 2021, 24 (2021) [Crossref] -
Kasieczka et al., Automating the ABCD method with machine learning
Phys. Rev. D 103, 035021 (2021) [Crossref] -
Mikuni et al., High-dimensional and permutation invariant anomaly detection
SciPost Phys. 16, 062 (2024) [Crossref] -
Yalcin Kuzu, Machine learning model performances for the Z boson mass
Eur. Phys. J. Plus 138, 41 (2023) [Crossref] -
Kasieczka et al., Quark-gluon tagging: Machine learning vs detector
SciPost Phys. 6, 069 (2019) [Crossref] -
Bollweg et al., Deep-learning jets with uncertainties and more
SciPost Phys. 8, 006 (2020) [Crossref] -
Albouy et al., Theory, phenomenology, and experimental avenues for dark showers: a Snowmass 2021 report
Eur. Phys. J. C 82, 1132 (2022) [Crossref] -
Datta et al., Automating the construction of jet observables with machine learning
Phys. Rev. D 100, 095016 (2019) [Crossref] -
Bernreuther et al., Casting a graph net to catch dark showers
SciPost Phys. 10, 046 (2021) [Crossref] -
Jawahar et al., Improving Variational Autoencoders for New Physics Detection at the LHC With Normalizing Flows
Front. Big Data 5, 803685 (2022) [Crossref] -
Govorkova et al., Autoencoders on field-programmable gate arrays for real-time, unsupervised new physics detection at 40 MHz at the Large Hadron Collider
Nat Mach Intell 4, 154 (2022) [Crossref] -
Aguilar-Saavedra et al., The minimal stealth boson: models and benchmarks
J. High Energ. Phys. 2019, 237 (2019) [Crossref] -
Baldi et al., How to GAN Higher Jet Resolution
SciPost Phys. 13, 064 (2022) [Crossref] -
De et al., Deep learning techniques for imaging air Cherenkov telescopes
Phys. Rev. D 107, 083026 (2023) [Crossref] -
Caron et al., Mixture-of-Theories training: can we find new physics and anomalies better by mixing physical theories?
J. High Energ. Phys. 2023, 4 (2023) [Crossref] -
Cheng et al., Invariant representation driven neural classifier for anti-QCD jet tagging
J. High Energ. Phys. 2022, 152 (2022) [Crossref] -
Gandrakota et al., Model selection and signal extraction using Gaussian Process regression
J. High Energ. Phys. 2023, 230 (2023) [Crossref] -
Aad et al., Anomaly detection search for new resonances decaying into a Higgs boson and a generic new particle
X
in hadronic final states using
s=13 TeV
pp
collisions with the ATLAS detector
Phys. Rev. D 108, 052009 (2023) [Crossref] -
Hallin et al., Classifying anomalies through outer density estimation
Phys. Rev. D 106, 055006 (2022) [Crossref] -
Dolan et al., Equivariant energy flow networks for jet tagging
Phys. Rev. D 103, 074022 (2021) [Crossref] -
Aguilar-Saavedra, Taming modeling uncertainties with mass unspecific supervised tagging
Eur. Phys. J. C 82, 270 (2022) [Crossref] -
Larkoski et al., Jet substructure at the Large Hadron Collider: A review of recent advances in theory and machine learning
Physics Reports 841, 1 (2020) [Crossref] -
Chakraborty et al., Interpretable deep learning for two-prong jet classification with jet spectra
J. High Energ. Phys. 2019, 135 (2019) [Crossref] -
Mullin et al., Does SUSY have friends? A new approach for LHC event analysis
J. High Energ. Phys. 2021, 160 (2021) [Crossref] -
Dillon et al., Better latent spaces for better autoencoders
SciPost Phys. 11, 061 (2021) [Crossref] -
Finke et al., Boosting mono-jet searches with model-agnostic machine learning
J. High Energ. Phys. 2022, 15 (2022) [Crossref] -
Cheng et al., Variational autoencoders for anomalous jet tagging
Phys. Rev. D 107, 016002 (2023) [Crossref] -
Heneka et al.,
472, 251 (2024) [Crossref] -
Gandrakota et al., Robust anomaly detection for particle physics using multi-background representation learning
Mach. Learn.: Sci. Technol. 5, 035082 (2024) [Crossref] -
Caron et al., Rare and Different: Anomaly Scores from a combination of likelihood and out-of-distribution models to detect new physics at the LHC
SciPost Phys. 12, 077 (2022) [Crossref] -
Flacke et al., Uncovering doubly charged scalars with dominant three-body decays using machine learning
J. High Energ. Phys. 2023, 9 (2023) [Crossref] -
Krause et al., Anomaly detection with flow-based fast calorimeter simulators
Phys. Rev. D 110, 035036 (2024) [Crossref] -
Kasieczka et al., The Machine Learning landscape of top taggers
SciPost Phys. 7, 014 (2019) [Crossref] -
Buss et al., What's anomalous in LHC jets?
SciPost Phys. 15, 168 (2023) [Crossref] -
Kim et al., The motivation and status of two-body resonance decays after the LHC Run 2 and beyond
J. High Energ. Phys. 2020, 30 (2020) [Crossref] -
D’Agnolo et al., Learning multivariate new physics
Eur. Phys. J. C 81, 89 (2021) [Crossref] -
Fraser et al., Challenges for unsupervised anomaly detection in particle physics
J. High Energ. Phys. 2022, 66 (2022) [Crossref] -
Metodiev et al., Anomaly detection in collider physics via factorized observables
Phys. Rev. D 110, 055012 (2024) [Crossref] -
Anzalone et al., Triggering dark showers with conditional dual auto-encoders
Mach. Learn.: Sci. Technol. 5, 035064 (2024) [Crossref] -
Blance et al., Adversarially-trained autoencoders for robust unsupervised new physics searches
J. High Energ. Phys. 2019, 47 (2019) [Crossref] -
Chigusa et al., Quantum simulations of dark sector showers
Physics Letters B 834, 137466 137466 (2022) [Crossref] -
Hallin et al., Resonant anomaly detection without background sculpting
Phys. Rev. D 107, 114012 (2023) [Crossref] -
Sahu et al., ML-based top taggers: Performance, uncertainty and impact of tower & tracker data integration
SciPost Phys. 17, 166 (2024) [Crossref] -
Beauchesne et al., Searching for periodic signals in kinematic distributions using continuous wavelet transforms
Eur. Phys. J. C 80, 192 (2020) [Crossref] -
Hao et al., Lorentz group equivariant autoencoders
Eur. Phys. J. C 83, 485 (2023) [Crossref] -
Nachman et al., Anomaly detection with density estimation
Phys. Rev. D 101, 075042 (2020) [Crossref] -
Bradshaw et al., Creating simple, interpretable anomaly detectors for new physics in jet substructure
Phys. Rev. D 106, 035014 (2022) [Crossref] -
Aarrestad et al., The Dark Machines Anomaly Score Challenge: Benchmark Data and Model Independent Event Classification for the Large Hadron Collider
SciPost Phys. 12, 043 (2022) [Crossref] -
Cohen et al., Jet substructure from dark sector showers
J. High Energ. Phys. 2020, 118 (2020) [Crossref] -
Aguilar-Saavedra et al., Mass Unspecific Supervised Tagging (MUST) for boosted jets
J. High Energ. Phys. 2021, 12 (2021) [Crossref] -
Amram et al., Tag N’ Train: a technique to train improved classifiers on unlabeled data
J. High Energ. Phys. 2021, 153 (2021) [Crossref] -
Krzyzanska et al., Simulation-based anomaly detection for multileptons at the LHC
J. High Energ. Phys. 2023, 61 (2023) [Crossref] -
Dillon et al., Uncovering latent jet substructure
Phys. Rev. D 100, 056002 (2019) [Crossref] -
Jiang et al., Detecting new physics as novelty — Complementarity matters
J. High Energ. Phys. 2022, 85 (2022) [Crossref] -
Freytsis et al., Anomaly detection in the presence of irrelevant features
J. High Energ. Phys. 2024, 220 (2024) [Crossref] -
Park et al., Neural embedding: learning the embedding of the manifold of physics data
J. High Energ. Phys. 2023, 108 (2023) [Crossref] -
Chekanov et al., Enhancing the hunt for new phenomena in dijet final states using anomaly detection filters at the high-luminosity large Hadron Collider
Eur. Phys. J. Plus 139, 237 (2024) [Crossref] -
Hashemi et al., Deep generative models for detector signature simulation: A taxonomic review
Reviews in Physics 12, 100092 100092 (2024) [Crossref] -
Dillon et al., Learning the latent structure of collider events
J. High Energ. Phys. 2020, 206 (2020) [Crossref] -
Aguilar-Saavedra et al., Multiphoton signals of a (96 GeV?) stealth boson
Eur. Phys. J. C 80, 403 (2020) [Crossref] -
Benkendorfer et al., Simulation-assisted decorrelation for resonant anomaly detection
Phys. Rev. D 104, 035003 (2021) [Crossref] -
Aguilar-Saavedra et al., Gradient boosting MUST taggers for highly-boosted jets
Eur. Phys. J. Plus 139, 1019 (2024) [Crossref] -
Kasieczka et al., Per-object systematics using deep-learned calibration
SciPost Phys. 9, 089 (2020) [Crossref] -
Bortolato et al., Bump hunting in latent space
Phys. Rev. D 105, 115009 (2022) [Crossref] -
Matchev et al., Finding wombling boundaries in LHC data with Voronoi and Delaunay tessellations
J. High Energ. Phys. 2020, 137 (2020) [Crossref] -
Aguilar-Saavedra et al., Exotic diboson $$Z'$$ decays in the U$$\mu \nu $$SSM
Eur. Phys. J. C 81, 805 (2021) [Crossref] -
Sengupta et al., Improving new physics searches with diffusion models for event observables and jet constituents
J. High Energ. Phys. 2024, 109 (2024) [Crossref] -
Letizia et al., Learning new physics efficiently with nonparametric methods
Eur. Phys. J. C 82, 879 (2022) [Crossref] -
Chakraborty et al., Neural network-based top tagger with two-point energy correlations and geometry of soft emissions
J. High Energ. Phys. 2020, 111 (2020) [Crossref] -
Ali et al., Elucidating the jet cross-section in pp and pPb collisions at sNN = 5.02TeV
Int. J. Mod. Phys. E 31, 2250102 (2022) [Crossref] -
Knapen et al., Perturbative benchmark models for a dark shower search program
Phys. Rev. D 103, 115013 (2021) [Crossref] -
Devlin et al., Diffusion model approach to simulating electron-proton scattering events
Phys. Rev. D 110, 016030 (2024) [Crossref] -
Mikuni et al., Online-compatible unsupervised nonresonant anomaly detection
Phys. Rev. D 105, 055006 (2022) [Crossref] -
Erdmann et al., Maximum performance of strange-jet tagging at hadron colliders
J. Inst. 16, P08039 (2021) [Crossref] -
Atkinson et al., IRC-Safe Graph Autoencoder for Unsupervised Anomaly Detection
Front. Artif. Intell. 5, 943135 (2022) [Crossref] -
Diefenbacher et al., CapsNets continuing the convolutional quest
SciPost Phys. 8, 023 (2020) [Crossref] -
Knapp et al., Adversarially Learned Anomaly Detection on CMS open data: re-discovering the top quark
Eur. Phys. J. Plus 136, 236 (2021) [Crossref] -
Bonilla et al., Jets and Jet Substructure at Future Colliders
Front. Phys. 10, 897719 (2022) [Crossref] -
Thaprasop et al., Unsupervised outlier detection in heavy-ion collisions
Phys. Scr. 96, 064003 (2021) [Crossref] -
Aad et al., Search for New Phenomena in Two-Body Invariant Mass Distributions Using Unsupervised Machine Learning for Anomaly Detection at
s=13 TeV
with the ATLAS Detector
Phys. Rev. Lett. 132, 081801 (2024) [Crossref] -
D’Agnolo et al., Learning new physics from an imperfect machine
Eur. Phys. J. C 82, 275 (2022) [Crossref] -
Ostdiek, Deep Set Auto Encoders for Anomaly Detection in Particle Physics
SciPost Phys. 12, 045 (2022) [Crossref] -
Komiske et al., Preserving new physics while simultaneously unfolding all observables
Phys. Rev. D 104, 076027 (2021) [Crossref] -
Dillon et al., Anomalies, representations, and self-supervision
SciPost Phys. Core 7, 056 (2024) [Crossref] -
Sengupta et al., CURTAINs flows for flows: Constructing unobserved regions with maximum likelihood estimation
SciPost Phys. 17, 046 (2024) [Crossref] -
Aguilar-Saavedra, Anomaly detection from mass unspecific jet tagging
Eur. Phys. J. C 82, 130 (2022) [Crossref] -
Dillon et al., A normalized autoencoder for LHC triggers
SciPost Phys. Core 6, 074 (2023) [Crossref] -
Oleksiyuk et al., Cluster Scanning: a novel approach to resonance searches
J. High Energ. Phys. 2024, 163 (2024) [Crossref] -
Dillon et al., Self-supervised anomaly detection for new physics
Phys. Rev. D 106, 056005 (2022) [Crossref] -
Herrero-Garcia et al., A semi-supervised approach to dark matter
searches in direct detection data with machine learning
J. Cosmol. Astropart. Phys. 2022, 039 (2022) [Crossref] -
Dreyer et al., Leveraging universality of jet taggers through transfer learning
Eur. Phys. J. C 82, 564 (2022) [Crossref] -
Dorigo et al., RanBox: anomaly detection in the copula space
J. High Energ. Phys. 2023, 8 (2023) [Crossref] -
Roche et al., Nanosecond anomaly detection with decision trees and real-time application to exotic Higgs decays
Nat Commun 15, 3527 (2024) [Crossref] -
Kasieczka et al., Anomaly detection under coordinate transformations
Phys. Rev. D 107, 015009 (2023) [Crossref] -
Yevick, Variational autoencoder analysis of Ising model statistical distributions and phase transitions
Eur. Phys. J. B 95, 56 (2022) [Crossref] -
Raine et al., CURTAINs for your sliding window: Constructing unobserved regions by transforming adjacent intervals
Front. Big Data 6, 899345 (2023) [Crossref] -
Belis et al., Machine learning for anomaly detection in particle physics
Reviews in Physics 12, 100091 100091 (2024) [Crossref] -
Barron et al., Unsupervised hadronic SUEP at the LHC
J. High Energ. Phys. 2021, 129 (2021) [Crossref] -
Bhattacherjee et al., Study of energy deposition patterns in hadron calorimeter for prompt and displaced jets using convolutional neural network
J. High Energ. Phys. 2019, 156 (2019) [Crossref] -
Nachman, A guide for deploying Deep Learning in LHC searches: How to achieve optimality and account for uncertainty
SciPost Phys. 8, 090 (2020) [Crossref] -
Bardhan et al., Unsupervised and lightly supervised learning in particle physics
Eur. Phys. J. Spec. Top. 233, 2559 (2024) [Crossref] -
Abdughani et al., Probing the triple Higgs boson coupling with machine learning at the LHC
Phys. Rev. D 104, 056003 (2021) [Crossref] -
Khosa et al., Anomaly Awareness
SciPost Phys. 15, 053 (2023) [Crossref]
Authors / Affiliation: mappings to Contributors and Organizations
See all Organizations.- 1 Theo Heimel,
- 1 Gregor Kasieczka,
- 1 Tilman Plehn,
- 1 Jennifer Thompson