Investigating ultrafast quantum magnetism with machine learning
Giammarco Fabiani, Johan H. Mentink
SciPost Phys. 7, 004 (2019) · published 5 July 2019
- doi: 10.21468/SciPostPhys.7.1.004
- Submissions/Reports
-
Abstract
We investigate the efficiency of the recently proposed Restricted Boltzmann Machine (RBM) representation of quantum many-body states to study both the static properties and quantum spin dynamics in the two-dimensional Heisenberg model on a square lattice. For static properties we find close agreement with numerically exact Quantum Monte Carlo results in the thermodynamical limit. For dynamics and small systems, we find excellent agreement with exact diagonalization, while for systems up to N=256 spins close consistency with interacting spin-wave theory is obtained. In all cases the accuracy converges fast with the number of network parameters, giving access to much bigger systems than feasible before. This suggests great potential to investigate the quantum many-body dynamics of large scale spin systems relevant for the description of magnetic materials strongly out of equilibrium.
Cited by 26
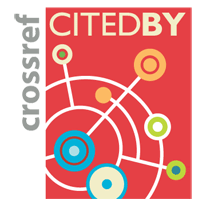
Ontology / Topics
See full Ontology or Topics database.Authors / Affiliation: mappings to Contributors and Organizations
See all Organizations.- Nederlandse Organisatie voor Wetenschappelijk Onderzoek / Netherlands Organisation for Scientific Research [NWO]
- Shell Exploration and Production Company (through Organization: Shell (Netherlands))
- Stichting voor Fundamenteel Onderzoek der Materie / Foundation For Fundamental Research On Matter [FOM]
- Stichting voor de Technische Wetenschappen (through Organization: Technologiestichting STW / Technology Foundation STW)