Reports of my demise are greatly exaggerated: $N$-subjettiness taggers take on jet images
Liam Moore, Karl Nordström, Sreedevi Varma, Malcolm Fairbairn
SciPost Phys. 7, 036 (2019) · published 24 September 2019
- doi: 10.21468/SciPostPhys.7.3.036
- Submissions/Reports
-
Abstract
We compare the performance of a convolutional neural network (CNN) trained on jet images with dense neural networks (DNNs) trained on n-subjettiness variables to study the distinguishing power of these two separate techniques applied to top quark decays. We find that they perform almost identically and are highly correlated once jet mass information is included, which suggests they are accessing the same underlying information which can be intuitively understood as being contained in 4-, 5-, 6-, and 8-body kinematic phase spaces depending on the sample. This suggests both of these methods are highly useful for heavy object tagging and provides a tentative answer to the question of what the image network is actually learning.
Cited by 25
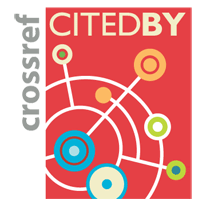
Ontology / Topics
See full Ontology or Topics database.Authors / Affiliations: mappings to Contributors and Organizations
See all Organizations.- 1 Liam Moore,
- 2 3 Karl Nordström,
- 4 Sreedevi Varma,
- 4 Malcolm Fairbairn
- 1 Université catholique de Louvain [UCL]
- 2 Laboratoire de Physique Théorique et Hautes Energies / Laboratory of Theoretical and High Energy Physics [LPTHE]
- 3 Nationaal instituut voor Subatomaire Fysica / National Institute for Subatomic Physics [NIKHEF]
- 4 King's College London [KCL]
- European Research Council [ERC]
- Fonds De La Recherche Scientifique - FNRS (FNRS) (through Organization: Fonds National de la Recherche Scientifique [FNRS])
- Nederlandse Organisatie voor Wetenschappelijk Onderzoek / Netherlands Organisation for Scientific Research [NWO]
- Science and Technology Facilities Council [STFC]