How to GAN LHC events
Anja Butter, Tilman Plehn, Ramon Winterhalder
SciPost Phys. 7, 075 (2019) · published 4 December 2019
- doi: 10.21468/SciPostPhys.7.6.075
- Submissions/Reports
-
Abstract
Event generation for the LHC can be supplemented by generative adversarial networks, which generate physical events and avoid highly inefficient event unweighting. For top pair production we show how such a network describes intermediate on-shell particles, phase space boundaries, and tails of distributions. In particular, we introduce the maximum mean discrepancy to resolve sharp local features. It can be extended in a straightforward manner to include for instance off-shell contributions, higher orders, or approximate detector effects.
TY - JOUR
PB - SciPost Foundation
DO - 10.21468/SciPostPhys.7.6.075
TI - How to GAN LHC events
PY - 2019/12/04
UR - https://scipost.org/SciPostPhys.7.6.075
JF - SciPost Physics
JA - SciPost Phys.
VL - 7
IS - 6
SP - 075
A1 - Butter, Anja
AU - Plehn, Tilman
AU - Winterhalder, Ramon
AB - Event generation for the LHC can be supplemented by generative adversarial networks, which generate physical events and avoid highly inefficient event unweighting. For top pair production we show how such a network describes intermediate on-shell particles, phase space boundaries, and tails of distributions. In particular, we introduce the maximum mean discrepancy to resolve sharp local features. It can be extended in a straightforward manner to include for instance off-shell contributions, higher orders, or approximate detector effects.
ER -
@Article{10.21468/SciPostPhys.7.6.075,
title={{How to GAN LHC events}},
author={Anja Butter and Tilman Plehn and Ramon Winterhalder},
journal={SciPost Phys.},
volume={7},
pages={075},
year={2019},
publisher={SciPost},
doi={10.21468/SciPostPhys.7.6.075},
url={https://scipost.org/10.21468/SciPostPhys.7.6.075},
}
Cited by 99
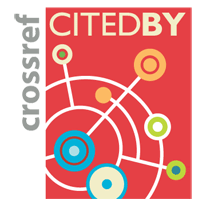
-
Chisholm et al., Non-parametric data-driven background modelling using conditional probabilities
J. High Energ. Phys. 2022, 1 (2022) [Crossref] -
Badger et al., Loop amplitudes from precision networks
SciPost Phys. Core 6, 034 (2023) [Crossref] -
Lu et al., Resolving extreme jet substructure
J. High Energ. Phys. 2022, 46 (2022) [Crossref] -
Das et al., How to understand limitations of generative networks
SciPost Phys. 16, 031 (2024) [Crossref] -
Bothmann et al., Exploring phase space with Neural Importance Sampling
SciPost Phys. 8, 069 (2020) [Crossref] -
Finke et al., Autoencoders for unsupervised anomaly detection in high energy physics
J. High Energ. Phys. 2021, 161 (2021) [Crossref] -
Carrazza et al., Lund jet images from generative and cycle-consistent adversarial networks
Eur. Phys. J. C 79, 979 (2019) [Crossref] -
Andreassen et al., Parameter estimation using neural networks in the presence of detector effects
Phys. Rev. D 103, 036001 (2021) [Crossref] -
Bellagente et al., How to GAN away detector effects
SciPost Phys. 8, 070 (2020) [Crossref] -
Larkoski, General analysis for observing quantum interference at colliders
Phys. Rev. D 105, 096012 (2022) [Crossref] -
Ackerschott et al., Returning CP-observables to the frames they belong
SciPost Phys. 17, 001 (2024) [Crossref] -
Badger et al., Using neural networks for efficient evaluation of high multiplicity scattering amplitudes
J. High Energ. Phys. 2020, 114 (2020) [Crossref] -
Danziger et al., Accelerating Monte Carlo event generation -- rejection sampling using neural network event-weight estimates
SciPost Phys. 12, 164 (2022) [Crossref] -
Chen et al., Improved neural network Monte Carlo simulation
SciPost Phys. 10, 023 (2021) [Crossref] -
Jinno et al., Machine learning Post-Minkowskian integrals
J. High Energ. Phys. 2023, 181 (2023) [Crossref] -
Baldi et al., How to GAN Higher Jet Resolution
SciPost Phys. 13, 064 (2022) [Crossref] -
Wang et al., A novel scenario in the semi-constrained NMSSM
J. High Energ. Phys. 2020, 78 (2020) [Crossref] -
Backes et al., How to GAN Event Unweighting
SciPost Phys. 10, 089 (2021) [Crossref] -
Maître et al., One-loop matrix element emulation with factorisation awareness
J. High Energ. Phys. 2023, 159 (2023) [Crossref] -
et al., Deep Generative Models for Fast Photon Shower Simulation in ATLAS
Comput Softw Big Sci 8, 7 (2024) [Crossref] -
Butter et al., Machine learning and LHC event generation
SciPost Phys. 14, 079 (2023) [Crossref] -
Aylett-Bullock et al., Optimising simulations for diphoton production at hadron colliders using amplitude neural networks
J. High Energ. Phys. 2021, 66 (2021) [Crossref] -
Elflein et al., Ultra-fast generation of air shower images for Imaging Air Cherenkov Telescopes using Generative Adversarial Networks
J. Inst. 19, P04010 (2024) [Crossref] -
Bieringer et al., Calomplification — the power of generative calorimeter models
J. Inst. 17, P09028 (2022) [Crossref] -
Nachman et al., Anomaly detection with density estimation
Phys. Rev. D 101, 075042 (2020) [Crossref] -
Bellagente et al., Understanding Event-Generation Networks via Uncertainties
SciPost Phys. 13, 003 (2022) [Crossref] -
Kansal et al., Evaluating generative models in high energy physics
Phys. Rev. D 107, 076017 (2023) [Crossref] -
Stienen et al., Phase space sampling and inference from weighted events with autoregressive flows
SciPost Phys. 10, 038 (2021) [Crossref] -
Isaacson, Generators and the (Accelerated) Future
J. Phys.: Conf. Ser. 2438, 012001 (2023) [Crossref] -
Gao et al., Event generation with normalizing flows
Phys. Rev. D 101, 076002 (2020) [Crossref] -
Maddrell-Mander et al., Studying the Potential of Graphcore® IPUs for Applications in Particle Physics
Comput Softw Big Sci 5, 8 (2021) [Crossref] -
Leigh et al., Faster diffusion model with improved quality for particle cloud generation
Phys. Rev. D 109, 012010 (2024) [Crossref] -
Bhattacherjee et al., Study of energy deposition patterns in hadron calorimeter for prompt and displaced jets using convolutional neural network
J. High Energ. Phys. 2019, 156 (2019) [Crossref] -
Araz et al., Quantum-inspired event reconstruction with Tensor Networks: Matrix Product States
J. High Energ. Phys. 2021, 112 (2021) [Crossref] -
Maître et al., Multi-variable integration with a neural network
J. High Energ. Phys. 2023, 221 (2023) [Crossref] -
Krause et al., Fast and accurate simulations of calorimeter showers with normalizing flows
Phys. Rev. D 107, 113003 (2023) [Crossref] -
Butter et al., How to GAN event subtraction
SciPost Phys. Core 3, 009 (2020) [Crossref] -
Kuttan et al., An equation-of-state-meter for CBM using PointNet
J. High Energ. Phys. 2021, 184 (2021) [Crossref] -
Chen et al., Deep learning jet substructure from two-particle correlations
Phys. Rev. D 101, 114025 (2020) [Crossref] -
Dillon et al., Learning the latent structure of collider events
J. High Energ. Phys. 2020, 206 (2020) [Crossref] -
Rousselot et al., Generative invertible quantum neural networks
SciPost Phys. 16, 146 (2024) [Crossref] -
Bravo-Prieto et al., Style-based quantum generative adversarial networks for Monte Carlo events
Quantum 6, 777 777 (2022) [Crossref] -
Howard et al., Learning to simulate high energy particle collisions from unlabeled data
Sci Rep 12, 7567 (2022) [Crossref] -
Alanazi et al., Machine learning-based event generator for electron-proton scattering
Phys. Rev. D 106, 096002 (2022) [Crossref] -
Leigh et al., PC-JeDi: Diffusion for particle cloud generation in high energy physics
SciPost Phys. 16, 018 (2024) [Crossref] -
Lai et al., Explainable machine learning of the underlying physics of high-energy particle collisions
Physics Letters B 829, 137055 137055 (2022) [Crossref] -
Ba et al., SHAPER: can you hear the shape of a jet?
J. High Energ. Phys. 2023, 195 (2023) [Crossref] -
et al., Challenges in Monte Carlo Event Generator Software for High-Luminosity LHC
Comput Softw Big Sci 5, 12 (2021) [Crossref] -
Nachman et al., Elsa: enhanced latent spaces for improved collider simulations
Eur. Phys. J. C 83, 843 (2023) [Crossref] -
Biscarat et al., End-to-End Jet Classification of Boosted Top Quarks with CMS Open Data
EPJ Web Conf. 251, 04030 (2021) [Crossref] -
Lifson et al., Calculating the primary Lund Jet Plane density
J. High Energ. Phys. 2020, 170 (2020) [Crossref] -
Ghosh et al., Towards a deep learning model for hadronization
Phys. Rev. D 106, 096020 (2022) [Crossref] -
Menary et al., Learning to discover: expressive Gaussian mixture models for multi-dimensional simulation and parameter inference in the physical sciences
Mach. Learn.: Sci. Technol. 3, 015021 (2022) [Crossref] -
Bellagente et al., Invertible networks or partons to detector and back again
SciPost Phys. 9, 074 (2020) [Crossref] -
Velasco et al.,
1395, 245 (2022) [Crossref] -
Capozi et al., Exploring anomalous couplings in Higgs boson pair production through shape analysis
J. High Energ. Phys. 2020, 91 (2020) [Crossref] -
Munoz et al., Boost invariant polynomials for efficient jet tagging
Mach. Learn.: Sci. Technol. 3, 04LT05 (2022) [Crossref] -
Butter et al., Generative networks for precision enthusiasts
SciPost Phys. 14, 078 (2023) [Crossref] -
Campbell et al., Event generators for high-energy physics experiments
SciPost Phys. 16, 130 (2024) [Crossref] -
Nachman, A guide for deploying Deep Learning in LHC searches: How to achieve optimality and account for uncertainty
SciPost Phys. 8, 090 (2020) [Crossref] -
Finke et al., Boosting mono-jet searches with model-agnostic machine learning
J. High Energ. Phys. 2022, 15 (2022) [Crossref] -
Khosa et al., A simple guide from machine learning outputs to statistical criteria in particle physics
SciPost Phys. Core 5, 050 (2022) [Crossref] -
Carrazza et al., Compressing PDF sets using generative adversarial networks
Eur. Phys. J. C 81, 530 (2021) [Crossref] -
Diefenbacher et al., DCTRGAN: improving the precision of generative models with reweighting
J. Inst. 15, P11004 (2020) [Crossref] -
Butter, Normalizing Flows for LHC Theory
J. Phys.: Conf. Ser. 2438, 012004 (2023) [Crossref] -
Hashemi et al., Deep generative models for detector signature simulation: A taxonomic review
Reviews in Physics 12, 100092 100092 (2024) [Crossref] -
Chen et al., Analysis-Specific Fast Simulation at the LHC with Deep Learning
Comput Softw Big Sci 5, 15 (2021) [Crossref] -
Dreyer et al., Quarks and gluons in the Lund plane
J. High Energ. Phys. 2022, 177 (2022) [Crossref] -
Felser et al., Quantum-inspired machine learning on high-energy physics data
npj Quantum Inf 7, 111 (2021) [Crossref] -
Tsai et al., Inverting cosmic ray propagation by convolutional neural networks
J. Cosmol. Astropart. Phys. 2022, 044 (2022) [Crossref] -
Matchev et al., OASIS: Optimal Analysis-Specific Importance Sampling for event generation
SciPost Phys. 10, 034 (2021) [Crossref] -
Agarwal et al., Explainable AI for ML jet taggers using expert variables and layerwise relevance propagation
J. High Energ. Phys. 2021, 208 (2021) [Crossref] -
Winterhalder et al., Targeting multi-loop integrals with neural networks
SciPost Phys. 12, 129 (2022) [Crossref] -
Butter et al., Two invertible networks for the matrix element method
SciPost Phys. 15, 094 (2023) [Crossref] -
Touranakou et al., Particle-based fast jet simulation at the LHC with variational autoencoders
Mach. Learn.: Sci. Technol. 3, 035003 (2022) [Crossref] -
Pata et al., MLPF: efficient machine-learned particle-flow reconstruction using graph neural networks
Eur. Phys. J. C 81, 381 (2021) [Crossref] -
Kasieczka et al., The LHC Olympics 2020 a community challenge for anomaly detection in high energy physics
Rep. Prog. Phys. 84, 124201 (2021) [Crossref] -
Velasco et al.,
, 372 (2020) [Crossref] -
Buhmann et al., CaloClouds II: ultra-fast geometry-independent highly-granular calorimeter simulation
J. Inst. 19, P04020 (2024) [Crossref] -
Soybelman et al., Set-conditional set generation for particle physics
Mach. Learn.: Sci. Technol. 4, 045036 (2023) [Crossref] -
Shyamsundar et al., Variance reduction via simultaneous importance sampling and control variates techniques using vegas
SciPost Phys. Codebases, 28 (2024) [Crossref] -
Matchev et al., Uncertainties associated with GAN-generated datasets in high energy physics
SciPost Phys. 12, 104 (2022) [Crossref] -
Hammad et al., Riemannian data preprocessing in machine learning to focus on QCD color structure
J. Korean Phys. Soc. 83, 235 (2023) [Crossref] -
Fraser et al., Challenges for unsupervised anomaly detection in particle physics
J. High Energ. Phys. 2022, 66 (2022) [Crossref] -
Chakraborty et al., Neural network-based top tagger with two-point energy correlations and geometry of soft emissions
J. High Energ. Phys. 2020, 111 (2020) [Crossref] -
Doglioni et al., Physics Inspired Deep Neural Networks for Top Quark Reconstruction
EPJ Web Conf. 245, 06029 (2020) [Crossref] -
Nachman et al., Neural resampler for Monte Carlo reweighting with preserved uncertainties
Phys. Rev. D 102, 076004 (2020) [Crossref] -
Hashemi et al., Ultra-high-granularity detector simulation with intra-event aware generative adversarial network and self-supervised relational reasoning
Nat Commun 15, 4916 (2024) [Crossref] -
Gao et al., i- flow: High-dimensional integration and sampling with normalizing flows
Mach. Learn.: Sci. Technol. 1, 045023 (2020) [Crossref] -
Deutschmann et al., Accelerating HEP simulations with Neural Importance Sampling
J. High Energ. Phys. 2024, 83 (2024) [Crossref] -
Alghamdi et al., Toward a generative modeling analysis of CLAS exclusive
2π
photoproduction
Phys. Rev. D 108, 094030 (2023) [Crossref] -
Xu et al., Generative machine learning for detector response modeling with a conditional normalizing flow
J. Inst. 19, P02003 (2024) [Crossref] -
Butter et al., GANplifying event samples
SciPost Phys. 10, 139 (2021) [Crossref] -
Amram et al., Tag N’ Train: a technique to train improved classifiers on unlabeled data
J. High Energ. Phys. 2021, 153 (2021) [Crossref] -
Maître et al., A factorisation-aware Matrix element emulator
J. High Energ. Phys. 2021, 66 (2021) [Crossref] -
Mehtar-Tani et al., Tagging boosted hadronic objects with dynamical grooming
Phys. Rev. D 102, 114013 (2020) [Crossref] -
Buhmann et al., Getting High: High Fidelity Simulation of High Granularity Calorimeters with High Speed
Comput Softw Big Sci 5, 13 (2021) [Crossref] -
Yallup et al., Exploring phase space with nested sampling
Eur. Phys. J. C 82, 678 (2022) [Crossref] -
Delgado et al., Unsupervised quantum circuit learning in high energy physics
Phys. Rev. D 106, 096006 (2022) [Crossref]
Ontology / Topics
See full Ontology or Topics database.Authors / Affiliation: mappings to Contributors and Organizations
See all Organizations.
Funders for the research work leading to this publication