Learning Summary Statistics for Bayesian Inference with Autoencoders
Carlo Albert, Simone Ulzega, Firat Ozdemir, Fernando Perez-Cruz, Antonietta Mira
SciPost Phys. Core 5, 043 (2022) · published 1 September 2022
- doi: 10.21468/SciPostPhysCore.5.3.043
- Submissions/Reports
-
Abstract
For stochastic models with intractable likelihood functions, approximate Bayesian computation offers a way of approximating the true posterior through repeated comparisons of observations with simulated model outputs in terms of a small set of summary statistics. These statistics need to retain the information that is relevant for constraining the parameters but cancel out the noise. They can thus be seen as thermodynamic state variables, for general stochastic models. For many scientific applications, we need strictly more summary statistics than model parameters to reach a satisfactory approximation of the posterior. Therefore, we propose to use the inner dimension of deep neural network based Autoencoders as summary statistics. To create an incentive for the encoder to encode all the parameter-related information but not the noise, we give the decoder access to explicit or implicit information on the noise that has been used to generate the training data. We validate the approach empirically on two types of stochastic models.
Cited by 1
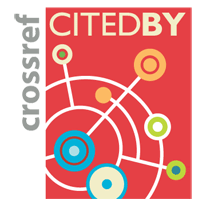
Authors / Affiliations: mappings to Contributors and Organizations
See all Organizations.- 1 Carlo Albert,
- 2 Simone Ulzega,
- 3 4 Firat Ozdemir,
- 4 Fernando Perez-Cruz,
- 5 6 Antonietta Mira
- 1 Eidgenössische Anstalt für Wasserversorgung, Abwasserreinigung und Gewässerschutz / Swiss Federal Institute of Aquatic Science and Technology [EAWAG]
- 2 Zürcher Hochschule für Angewandte Wissenschaften / Zurich University of Applied Sciences [ZHAW]
- 3 Eidgenössische Technische Hochschule Zürich / Swiss Federal Institute of Technology in Zurich (ETH) [ETH Zurich]
- 4 Swiss Data Science Center [SDSC]
- 5 Università degli Studi dell'Insubria / University of Insubria
- 6 Università della Svizzera italiana [USI]