Conditional generative models for sampling and phase transition indication in spin systems
Japneet Singh, Mathias S. Scheurer, Vipul Arora
SciPost Phys. 11, 043 (2021) · published 30 August 2021
- doi: 10.21468/SciPostPhys.11.2.043
- Submissions/Reports
-
Abstract
In this work, we study generative adversarial networks (GANs) as a tool to learn the distribution of spin configurations and to generate samples, conditioned on external tuning parameters or other quantities associated with individual configurations. For concreteness, we focus on two examples of conditional variables---the temperature of the system and the energy of the samples. We show that temperature-conditioned models can not only be used to generate samples across thermal phase transitions, but also be employed as unsupervised indicators of transitions. To this end, we introduce a GAN-fidelity measure that captures the model’s susceptibility to external changes of parameters. The proposed energy-conditioned models are integrated with Monte Carlo simulations to perform over-relaxation steps, which break the Markov chain and reduce auto-correlations. We propose ways of efficiently representing the physical states in our network architectures, e.g., by exploiting symmetries, and to minimize the correlations between generated samples. A detailed evaluation, using the two-dimensional XY model as an example, shows that these incorporations bring in considerable improvements over standard machine-learning approaches. We further study the performance of our architectures when no training data is provided near the critical region.
Cited by 13
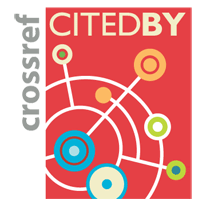
Authors / Affiliations: mappings to Contributors and Organizations
See all Organizations.- 1 Japneet Singh,
- 2 3 Mathias S. Scheurer,
- 1 Vipul Arora
- 1 भारतीय प्रौद्योगिकी संस्थान कानपुर / Indian Institute of Technology Kanpur [IITK]
- 2 Harvard University
- 3 Institut für Theoretische Physik / Institute for Theoretical Physics, University of Innsbruck [ITP]