Reconstructing partonic kinematics at colliders with machine learning
David F. Rentería Estrada, Roger J. Hernández-Pinto, German F. R. Sborlini, Pia Zurita
SciPost Phys. Core 5, 049 (2022) · published 31 October 2022
- doi: 10.21468/SciPostPhysCore.5.4.049
- Submissions/Reports
-
Abstract
In the context of high-energy physics, a reliable description of the parton-level kinematics plays a crucial role for understanding the internal structure of hadrons and improving the precision of the calculations. In proton-proton collisions, this represents a challenging task since extracting such information from experimental data is not straightforward. With this in mind, we propose to tackle this problem by studying the production of one hadron and a direct photon in proton-proton collisions, including up to Next-to-Leading Order Quantum Chromodynamics and Leading-Order Quantum Electrodynamics corrections. Using Monte-Carlo integration, we simulate the collisions and analyze the events to determine the correlations among measurable and partonic quantities. Then, we use these results to feed three different Machine Learning algorithms that allow us to find the momentum fractions of the partons involved in the process, in terms of suitable combinations of the final state momenta. Our results are compatible with previous findings and suggest a powerful application of Machine-Learning to model high-energy collisions at the partonic-level with high-precision.
Cited by 1
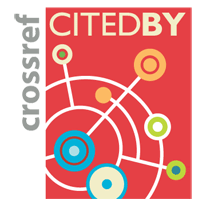
Authors / Affiliations: mappings to Contributors and Organizations
See all Organizations.- 1 David Rentería,
- 1 Roger J. Hernández-Pinto,
- 2 3 German Sborlini,
- 4 Pia Zurita
- 1 Universidad Autónoma de Sinaloa / Autonomous University of Sinaloa [UAS]
- 2 Deutsches Elektronen-Synchrotron / Deutsche Elektronen-Synchrotron DESY [DESY]
- 3 Universidad de Salamanca / University of Salamanca
- 4 Universität Regensburg / University of Regensburg
- Consejo Nacional de Ciencia y Tecnología [CONACYT]
- Deutsche Forschungsgemeinschaft / German Research FoundationDeutsche Forschungsgemeinschaft [DFG]
- European Cooperation in Science and Technology
- Horizon 2020 (through Organization: European Commission [EC])
- Ministerio de Ciencia e Innovación
- Universidad Autónoma de Sinaloa
- Universidad de Salamanca