A simple guide from machine learning outputs to statistical criteria in particle physics
Charanjit Kaur Khosa, Veronica Sanz, Michael Soughton
SciPost Phys. Core 5, 050 (2022) · published 2 November 2022
- doi: 10.21468/SciPostPhysCore.5.4.050
- Submissions/Reports
-
Abstract
In this paper we propose ways to incorporate Machine Learning training outputs into a study of statistical significance. We describe these methods in supervised classification tasks using a CNN and a DNN output, and unsupervised learning based on a VAE. As use cases, we consider two physical situations where Machine Learning are often used: high-$p_T$ hadronic activity, and boosted Higgs in association with a massive vector boson.
Cited by 2
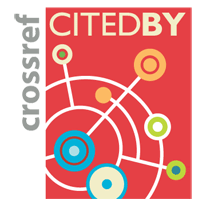
Authors / Affiliations: mappings to Contributors and Organizations
See all Organizations.- 1 University of Bristol
- 2 Universitat de València / University of Valencia [UV]
- 3 University of Sussex
Funders for the research work leading to this publication