Exploring unsupervised top tagging using Bayesian inference
Ezequiel Alvarez, Manuel Szewc, Alejandro Szynkman, Santiago A. Tanco, Tatiana Tarutina
SciPost Phys. Core 6, 046 (2023) · published 28 June 2023
- doi: 10.21468/SciPostPhysCore.6.2.046
- Submissions/Reports
-
Abstract
Recognizing hadronically decaying top-quark jets in a sample of jets, or even its total fraction in the sample, is an important step in many LHC searches for Standard Model and Beyond Standard Model physics as well. Although there exists outstanding top-tagger algorithms, their construction and their expected performance rely on Montecarlo simulations, which may induce potential biases. For these reasons we develop two simple unsupervised top-tagger algorithms based on performing Bayesian inference on a mixture model. In one of them we use as the observed variable a new geometrically-based observable $\tilde{A}_{3}$, and in the other we consider the more traditional $\tau_{3}/\tau_{2}$ $N$-subjettiness ratio, which yields a better performance. As expected, we find that the unsupervised tagger performance is below existing supervised taggers, reaching expected Area Under Curve AUC $\sim 0.80-0.81$ and accuracies of about 69\% $-$ 75\% in a full range of sample purity. However, these performances are more robust to possible biases in the Montecarlo that their supervised counterparts. Our findings are a step towards exploring and considering simpler and unbiased taggers.
Cited by 2
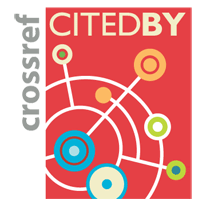
Ontology / Topics
See full Ontology or Topics database.Authors / Affiliations: mappings to Contributors and Organizations
See all Organizations.- 1 Ezequiel Alvarez,
- 2 Manuel Szewc,
- 3 Alejandro Szynkman,
- 3 Santiago A. Tanco,
- 1 Tatiana Tarutina
- 1 Consejo Nacional de Investigaciones Científicas y Técnicas / National Scientific and Technical Research Council [CONICET]
- 2 University of Cincinnati [UC]
- 3 Universidad Nacional de La Plata / National University of La Plata [UNLP]