Predicting quantum potentials by deep neural network and metropolis sampling
Rui Hong, Peng-Fei Zhou, Bin Xi, Jie Hu, An-Chun Ji, Shi-Ju Ran
SciPost Phys. Core 4, 022 (2021) · published 13 September 2021
- doi: 10.21468/SciPostPhysCore.4.3.022
- Submissions/Reports
-
Abstract
The hybridizations of machine learning and quantum physics have caused essential impacts to the methodology in both fields. Inspired by quantum potential neural network, we here propose to solve the potential in the Schrodinger equation provided the eigenstate, by combining Metropolis sampling with deep neural network, which we dub as Metropolis potential neural network (MPNN). A loss function is proposed to explicitly involve the energy in the optimization for its accurate evaluation. Benchmarking on the harmonic oscillator and hydrogen atom, MPNN shows excellent accuracy and stability on predicting not just the potential to satisfy the Schrodinger equation, but also the eigen-energy. Our proposal could be potentially applied to the ab-initio simulations, and to inversely solving other partial differential equations in physics and beyond.
Cited by 3
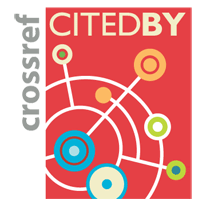
Authors / Affiliations: mappings to Contributors and Organizations
See all Organizations.- 1 Rui Hong,
- 1 Peng-Fei Zhou,
- 2 Bin Xi,
- 1 Jie Hu,
- 1 An-Chun Ji,
- 1 Shi-Ju Ran